Aug 5, 2020
Models can help flatten your business losses curve
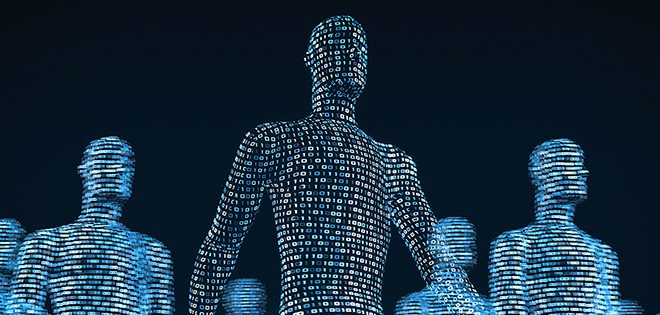
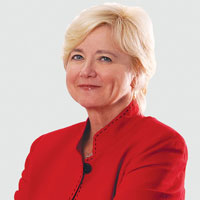
Data Science | SFBJ
Reprinted from South Florida Business Journal.
As the curve of Covid-19 cases rose, businesses’ losses also increased. And throughout this pandemic, we have heard more about predictive modeling than most of us will ever understand.
I was trying to explain to one of my friends that no model can predict the future with certainty. After a few questions, she said: “Oh, it’s like predicting the weather.” Weather forecasters have sophisticated technology and hundreds of years of historical information, but things change. And, if the variables change, their predictions have to change. Like any model, weather forecasts provide a percent of confidence, and we adjust our lives by their predictions.
Both virus models and weather predictions impact our human behavior—and human behavior is what we try to predict when we evaluate business performance. This is particularly true for marketing. And in light of the current situation, last year’s marketing predictive models need to be recalibrated.
Marketing data since January can help
To give you guidance on a losses curve, you can probably compare January and February 2020 data to March, April and May 2020. According to modeling expert Andrew Russo, those five months can potentially give you the foundation to determine a path to how fast your losses curve can flatten. Without current data, the models can’t be accurate. We saw that demonstrated with Covid-19 models that became more accurate as more data was collected, he explained.
“It seems to me,” Russo says, “that it’s initially going to be up to consumers versus business marketers to create the demand that results in consumer buying confidence. And that demand can help determine how fast businesses can get back to some level of profitability. Consumers drive the business curve, whether you are a B2C or B2B organization.” And that gets back to the importance of marketing data.
Models for purchase probability
A predictive model can also be built to calculate probability for prospects to purchase. One recent model’s test results, based on purchase data, indicated 85% of the sales would come from prospects with at least a 30% probability to purchase. Using this model to target customers, the purchase rate exceeded past results by 70%, while the cost per acquisition dropped by 30%. That’s a significant margin increase that goes directly to a business’s bottom line.
“A model is nothing without data that supports the business objectives that spawned the modeling project,” Russo advises. Most B2C companies have troves of data. If you’re keeping your data in a customer data platform, it will lead to more accurate model performance.
Keep common modeling errors from foiling your efforts
There are three main mistakes that can cause models to fail. First, if the data used to build the model is incomplete, biased or of poor quality. Second, if the model was built to address a specific objective, but then used for a different purpose. And finally, if the model’s design was not closely aligned with well-defined business objectives.
These mistakes will likely not occur if the predictive modeler has experience in building and implementing models, and has a clear understanding of the business goals and objectives to which the models must align.
Avoid rain on your parade
It is vital to have models that can segment target markets in several ways, including near-term and lifetime value, loyalty and retention. Applying these models will give any business a clear view on how and where to grow sales and focus marketing efforts.
The most important thing to remember about modeling is that it helps you manage downside and waste. Just like a forecast helps you avoid rain on your parade, a model can help you focus your marketing budget where it will make the most impact. It’s a great way to flatten your losses curve and improve your return on marketing—definitely a competitive advantage.